Immagini AI di te stesso in MENO DI 10 secondi Simili a InstantID (ma è FLUX) PuLID: Personalizzazione ID Pure e Lightning tramite allineamento contrastivo
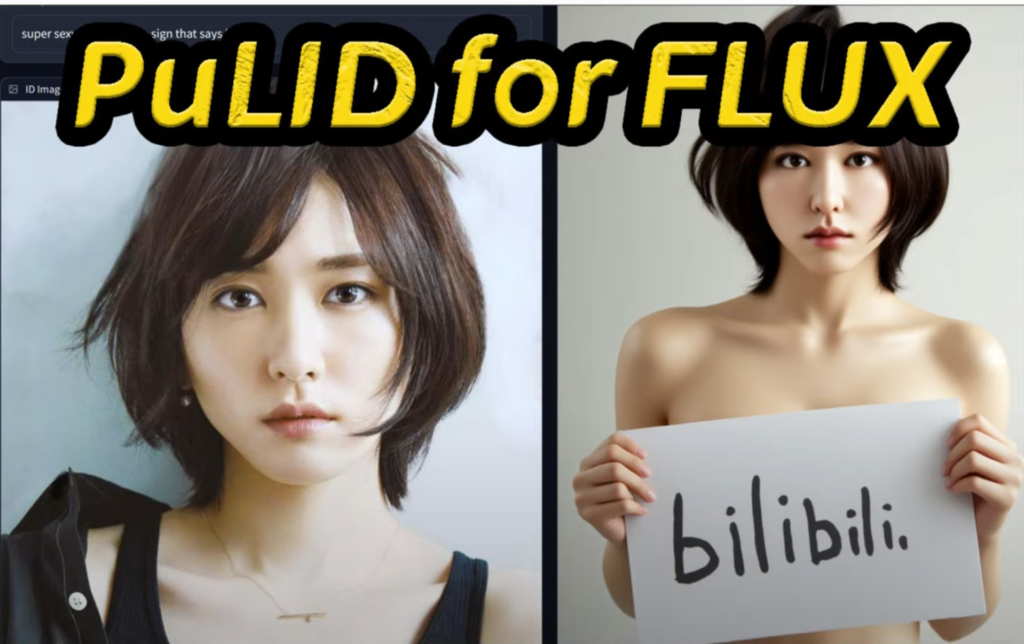
Introduzione
PuLID è un innovativo metodo di personalizzazione ID senza sintonizzazione progettato per la generazione di testo in immagini. Incorporando un ramo Lightning T2I insieme a uno standard di diffusione, PuLID introduce sia la perdita di allineamento contrastivo sia la perdita di ID accurata, riducendo al minimo l'interruzione del modello originale e garantendo un'elevata fedeltà ID. Gli esperimenti dimostrano che PuLID raggiunge prestazioni superiori sia in termini di fedeltà ID che di modificabilità. Inoltre, una caratteristica degna di nota di PuLID è che gli elementi dell'immagine (ad esempio, sfondo, illuminazione, composizione e stile) rimangono il più coerenti possibile prima e dopo l'inserimento dell'ID.
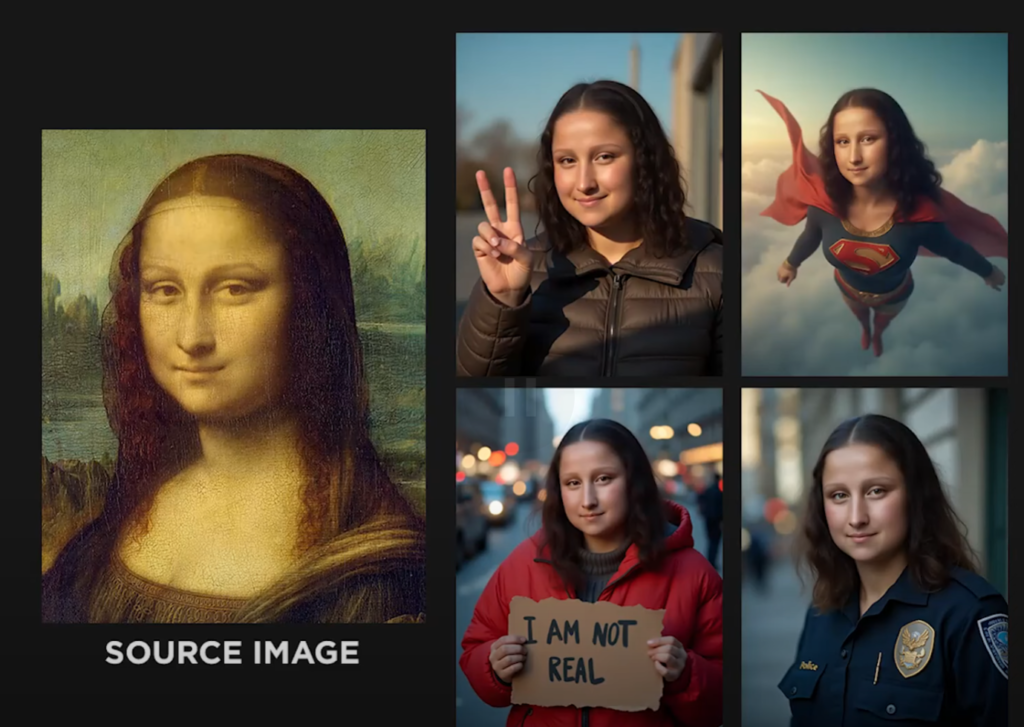
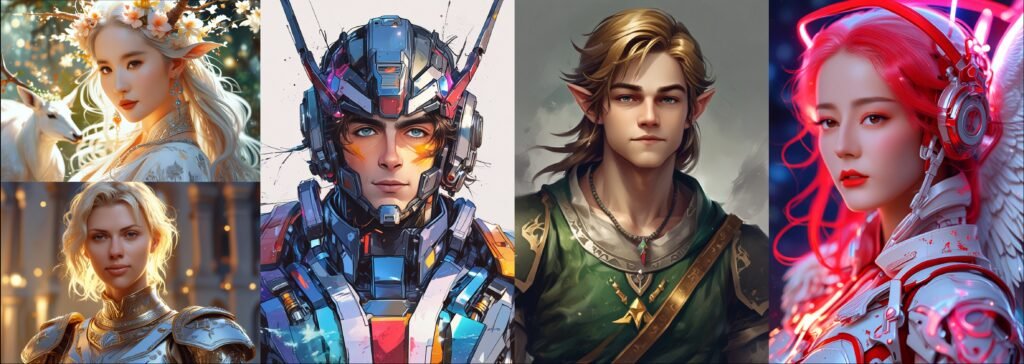
Tentativo PuLID per FLUX Gratuito
Cosa sta succedendo su Twitter riguardo Pulid Flux?
🚀Progetto numero 1 – PuLID-FLUX🔥
— ManuAGI 🤖 – ( ManuIn ) (@ManuAGI01) 17 settembre 2024
"Uno strumento di personalizzazione dell'identità personalizzata"#AI #HuggingFace #TechInnovazione #AIArte Modello di diffusione #D Modello #FLUX #AICodifica #CreativeAI #PuLIDFLUX foto.twitter.com/DxpZNEXZ4v
PULID per Flux:https://t.co/eejy2TeMZ3 https://t.co/XUc2HTm2sj foto.twitter.com/G35Zibt2Vn
—Luis C (@lucataco93) 13 settembre 2024
Aggiornamento PuLID-FLUX-v0.9.0 ⚙️.
— Lepre AI (@harecrypta_ai) 18 settembre 2024
Ho avvitato un nuovo personalizzatore PuLID a #Flusso 🧬.
Sembra così fantastico 🤩 foto.twitter.com/9e09lffXBk
Ora è possibile generare coerenza di carattere da una sola immagine usando Flux Pulid. Puoi generare immagini di un personaggio in abiti e scenari di illuminazione completamente diversi. Ti mostrerò i pro e i contro. https://t.co/vEHbnKPTbh Godere! #AI #Flusso #FluxPulito foto.twitter.com/I5G5gsqmml
— Travis Davids (@MrDavids1) 17 settembre 2024
「PuLID con FLUX」イケメンのランサーVer
— Laboratori di intelligenza artificiale Maki@Sunwood. (@hAru_mAki_ch) 16 settembre 2024
—-
Un bel lanciere da un mondo fantasy oscuro, catturato in uno stile cinematografico da 35 mm. Il lanciere si erge fiducioso con un'aura misteriosa, rivestito di un'armatura scura e intricata con una lunga lancia al fianco. Il suo viso è sorprendentemente bello, con… foto.twitter.com/XnLCAXudkV
PuLID per FLUX
— Grado (@Gradio) 17 settembre 2024
🔥Fornisce una soluzione di personalizzazione dell'ID senza necessità di messa a punto utilizzando FLUX-dev.
Questo progetto Gradio-first è di tendenza su GitHub! Congratulazioni a Yanze Wu e al team 👏
Scopri come giocare con il modello Pulid-FLUX a livello locale: https://t.co/5wgANTxhLa
Gioca con il… foto.twitter.com/ADjZDCtWMT
PuLIDFlux版本, 人脸还原效果还挺不错.
—Gorden Sun (@Gorden_Sun) 13 settembre 2024
Pagina inizialehttps://t.co/S1XAzReW24,
In questo articolo ti mostreremo:https://t.co/4bq73kk8rK
图1是原图,234是生成的 foto.twitter.com/qln6TDFHt2
Metodi
- Allineamento contrastivo: Tramite la perdita di allineamento contrastivo e la perdita di ID, PuLID inserisce le informazioni di ID senza influenzare il comportamento del modello originale.
- Ramo Lightning T2I: Introduce un ramo Lightning T2I che utilizza tecniche di campionamento rapido per generare immagini di alta qualità a partire da rumore puro.
- Ottimizzazione della perdita di ID: Ottimizza la perdita di ID in un'impostazione più accurata per migliorare la somiglianza degli ID.
Esperimenti
- Confronto quantitativo: Valuta la fedeltà dell'ID utilizzando la similarità del coseno dell'ID, dimostrando che PuLID supera i metodi esistenti in tutti i set di test e modelli di base.
- Confronto qualitativo: PuLID consente di ottenere un'elevata somiglianza degli ID, interrompendo al contempo il modello originale e riproducendone accuratamente l'illuminazione, lo stile e il layout.
Contributi
- Propone un metodo senza ottimizzazione, PuLID, che preserva un'elevata similarità degli ID, mitigando al contempo l'impatto sul comportamento del modello originale.
- Introduce un ramo Lightning T2I accanto al ramo di diffusione regolare, incorporando la perdita di allineamento contrastivo e la perdita di ID per ridurre al minimo la contaminazione delle informazioni di ID sul modello originale, garantendo al contempo la fedeltà.
- Gli esperimenti dimostrano che PuLID raggiunge prestazioni all'avanguardia in termini di fedeltà dell'ID e modificabilità ed è meno invasivo per il modello, rendendolo più flessibile per le applicazioni pratiche.